The economic impact of AI in healthcare is reshaping how we think about medical costs, efficiency, and patient care. As a startup working in this dynamic space, we’re witnessing a transformation that goes far beyond just automating tasks. Healthcare providers, from small clinics to major hospital networks, are grappling with rising costs, staff shortages, and increasing patient demands.
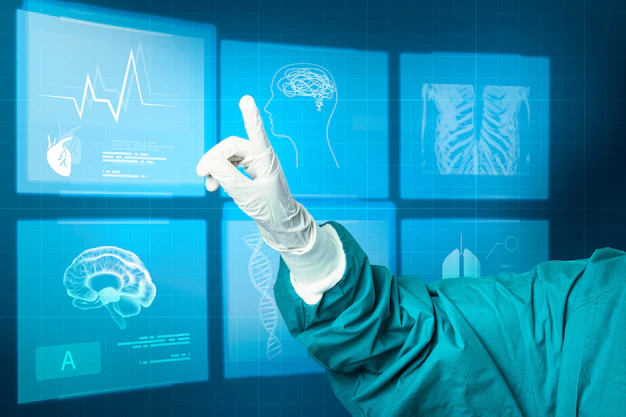
Meanwhile, AI technologies are emerging as potential solutions to these long-standing challenges. In this post, we’ll explore how AI is influencing healthcare economics, drawing from industry research and market analyses. While we’re still in the early stages of this revolution, the numbers and trends paint an interesting picture of where healthcare is heading.
Table of Contents
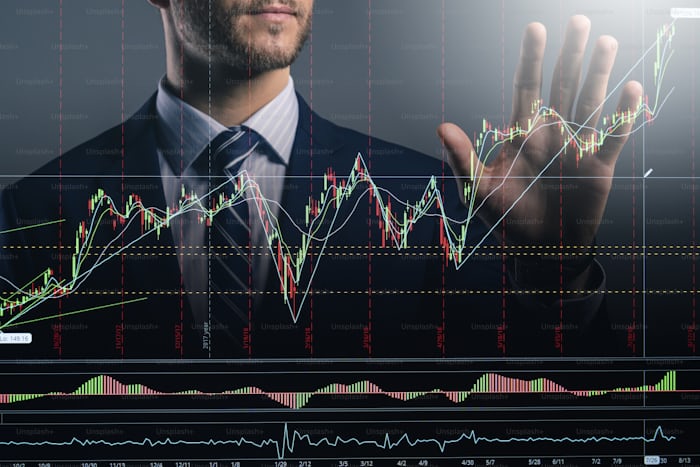
Breaking Down the Numbers: Market Analysis of AI-Powered Diagnostics
The global market for AI in medical diagnostics tells a compelling story about where healthcare is heading. According to recent market research, the AI diagnostics sector was valued at approximately $3.7 billion in 2020, with projections showing growth to reach nearly $67 billion by 2027. But what’s driving these numbers? The most significant impact comes from medical imaging, where AI is helping radiologists work more efficiently.
For instance, traditional chest X-ray analysis can take radiologists several minutes per image. AI-assisted screening can flag potential abnormalities in seconds, allowing radiologists to prioritize urgent cases and see more patients. Studies from major medical centers suggest this can reduce reading time by up to 30%.
Cost savings appear in multiple areas:
- Reduced time per diagnosis.
- Fewer unnecessary follow-up tests.
- Earlier detection of conditions, leading to more cost-effective treatment paths.
- Lower error rates in routine screenings.
- However, it’s important to note that AI isn’t replacing human expertise – it’s augmenting it.
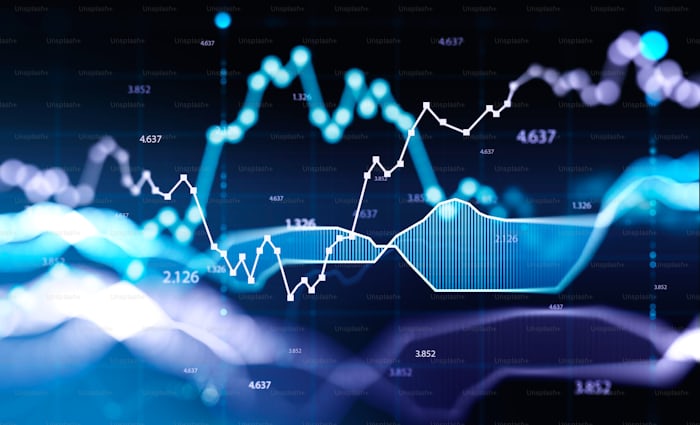
While the initial investment in AI diagnostic tools can be substantial, ranging from $100,000 to several million dollars depending on the system’s scope, healthcare providers are reporting positive returns within 18-24 months of implementation.
Real-world implementations at major hospitals have shown promising results. For example, the UK’s National Health Service found that AI-assisted breast cancer screening could reduce radiologist workload by up to 88% for normal cases, allowing specialists to focus on more complex cases requiring human expertise.
These numbers paint a picture of a technology that’s not just promising but is already delivering tangible economic benefits in real healthcare settings. The key is understanding where AI can provide the most value in your specific diagnostic workflow.
Scaling Care Without Scaling Costs: How AI Could Transform Patient Triage
Africa
In Africa, where the World Health Organization reports a ratio of about 1 doctor per 5,000 patients (compared to the recommended 1:1,000), efficient patient triage isn’t just about convenience – it’s about survival. This severe shortage of healthcare workers makes AI-powered triage solutions particularly relevant for the continent.
Several promising pilot programs and early implementations show how AI could help:
Mobile-First Solutions
Africa’s high mobile phone penetration (around 80%) creates a unique opportunity. Simple SMS or app-based AI triage tools can help people determine whether they need to make the often-lengthy journey to a healthcare facility. This saves both patients’ time and healthcare resources.
Community Health Worker Support
AI tools can support community health workers (CHWs) who are often the first point of contact for patients in rural areas. By providing decision support through basic smartphones, these tools can help CHWs:
- Assess symptoms more accurately.
- Prioritize cases effectively.
- Make better-informed referral decisions.
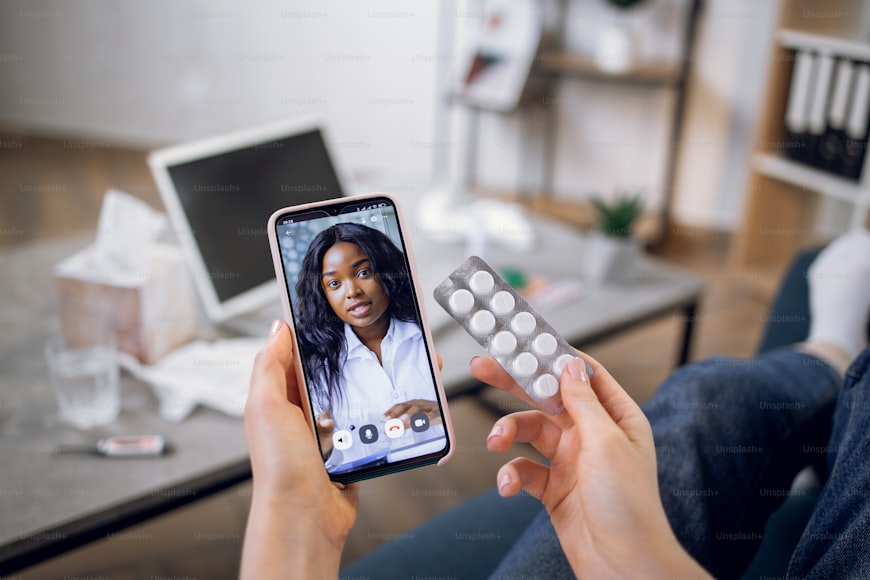
Cost Benefits for Healthcare Systems
The economic advantages are particularly relevant in resource-constrained settings:
- Reduced unnecessary hospital visits.
- Better allocation of limited specialist time.
- More efficient use of medical supplies and equipment.
- Lower transportation costs for patients.
Real-World Implementation
In Rwanda, several clinics are piloting AI-powered triage tools that help nurses assess patient urgency levels. Early data suggests these systems can help facilities handle up to 20% more patients daily without additional staff.
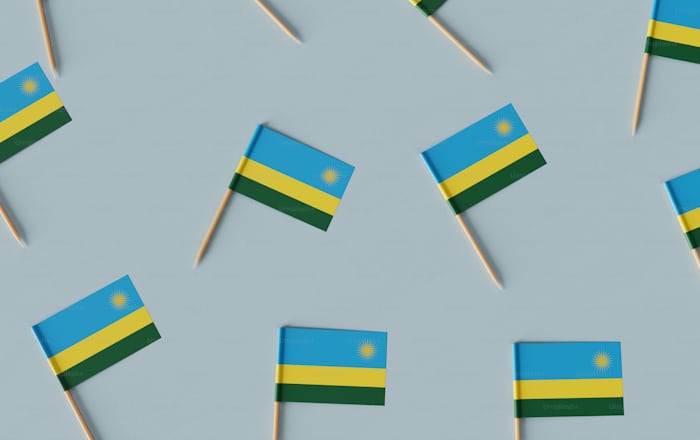
Challenges to Consider
While promising, implementing AI triage in Africa faces several hurdles, that are surmountable, namely:
- Limited internet connectivity in rural areas(this might change with the increasing adoption of Starlink, as a major internet provider on the continent, following reduced costs).
- Need for solutions that work in multiple local languages.
- Initial setup and training costs.
- Integration with existing healthcare systems
The key to success lies in developing solutions that are:
- Affordable and sustainable.
- Adaptable to local contexts.
- Usable with basic technology.
- Supportive of existing healthcare workflows etc
The potential for AI to help scale care in Africa is significant, but it’s crucial to approach implementation with a deep understanding of local contexts and challenges. The goal isn’t to replace human healthcare workers but to help them work more efficiently and serve more patients effectively.
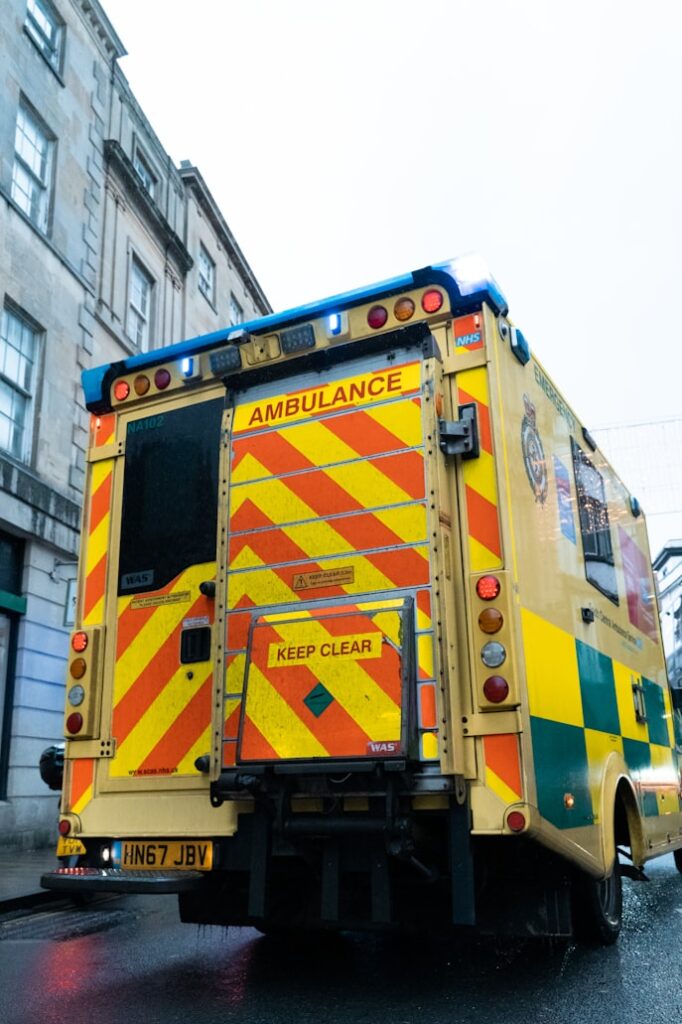
Globally
The global healthcare system faces a universal challenge: managing increasing patient loads while controlling costs. From emergency departments in New York to primary care clinics in London, AI-powered triage is emerging as a key solution for this healthcare puzzle.
Digital Front Doors
Modern AI triage often begins before patients even reach a healthcare facility:
- Virtual symptom checkers help patients decide if they need immediate care.
- Chatbots guide patients to appropriate care levels (urgent care vs. emergency room).
- Smart scheduling systems prioritize appointments based on symptom severity.
Emergency Department Impact
EDs worldwide are seeing promising results:
- Major hospitals report 15-20% reduction in waiting times.
- Better identification of high-risk patients who need immediate attention.
- Reduced costs associated with unnecessary emergency visits.
- More efficient allocation of medical staff.
Primary Care Evolution
In primary care settings, AI triage tools are showing significant benefits:
– Automated pre-visit screening- Risk stratification for chronic disease management- More efficient scheduling based on patient needs- Reduced administrative burden on medical staff
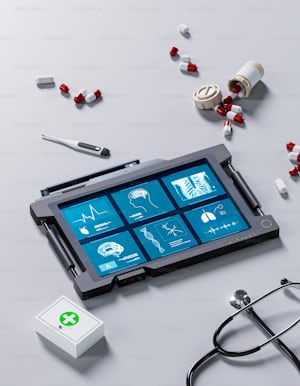
Economic BenefitsThe financial impact appears in multiple areas:
- Lower operational costs through better resource allocation
- Reduced unnecessary specialist referrals
- More efficient use of medical staff time
- Decreased liability risks through standardized assessment.
Real-World Implementation
- NHS (UK): AI triage systems helped reduce non-emergency ED visits by up to 20%.
- Kaiser Permanente (US): Remote triage tools decreased in-person visits while maintaining care quality
- Singapore Health Services: AI-powered triage reduced average waiting times by 30%.
Challenges in Implementation
- Initial investment costs.
- Staff training requirements.
- Integration with existing electronic health records.
- Privacy and security concerns.
- Need for continuous system updates and maintenance.
Successful AI triage systems typically need:
- Integration with electronic health records.
- Secure communication channels.
- User-friendly interfaces for both staff and patients.
- Robust data protection measures.
- Regular updates based on new medical guidelines.
Future Directions
The field is evolving toward:
- More sophisticated predictive analytics.
- Better integration with telehealth services.
- Enhanced personalization based on patient history.
- Improved multilingual capabilities.
- Greater use of remote monitoring data.
The key to successful implementation lies in finding the right balance between automation and human touch. While AI can handle routine triage tasks efficiently, human medical professionals remain essential for complex cases and final decision-making. Looking ahead, the integration of AI in triage isn’t just about managing patient flow – it’s about transforming how healthcare systems identify, prioritize, and respond to patient needs while maintaining cost efficiency.
From Pilot to Profit: The True Implementation Costs of Healthcare AI
The journey from deciding to implement AI to seeing actual returns is often more complex than vendors’ glossy presentations suggest.
Let’s break down the real costs and considerations that healthcare organizations need to understand.
Initial Investment Areas
1. Technology Infrastructure
- Hardware upgrades or cloud computing resources.
- Data storage and processing capabilities.
- Security system enhancements.
- Integration with existing systems
2. Human Capital Costs
- Staff training programs.
- New technical roles (data scientists, AI specialists)
- Project management resources
- Change management specialists
3. Data Preparation
- Data cleaning and standardization
- Legacy system integration
- Compliance and security measures
- Documentation and protocol development
Hidden Costs to Consider
- System downtime during implementation
- Productivity dips during the learning curve
- Regular software updates and maintenance
- Ongoing staff training and support
- Legal and compliance consulting
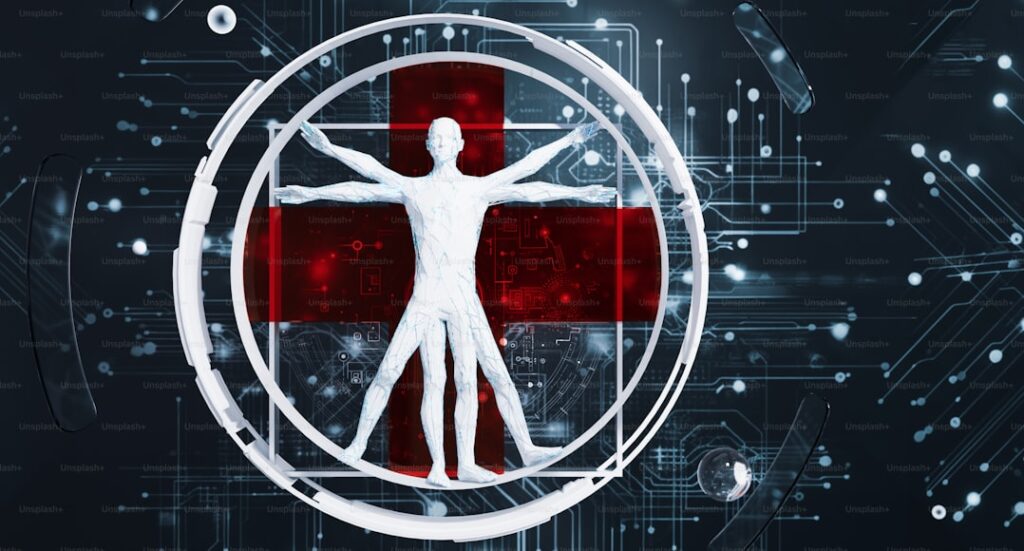
Timeline to ROI
Most healthcare organizations report:
- 6-12 months for initial implementation.
- 18-24 months to reach positive ROI.
- 3-5 years for full system maturity.
Success Factors That Impact Costs
- Clear use case definition.
- Strong change management.
- Robust staff training programs.
- Phased implementation approach.
- Regular performance monitoring
Risk Mitigation Strategies
- Starting with pilot programs.
- Choosing scalable solutions.
- Building in contingency budgets.
- Maintaining parallel systems initially..
- Regular stakeholder communication.
Measuring Success
Key metrics to track:
- Time saved per procedure/process.
- Error rate reduction.
- Patient satisfaction scores.
- Staff adoption rates.
- Cost per patient.
- Resource utilization improvements
The reality is that implementing healthcare AI is a significant investment that requires careful planning and realistic expectations. While the long-term benefits can be substantial, organizations need to be prepared for both the visible and hidden costs along the way.
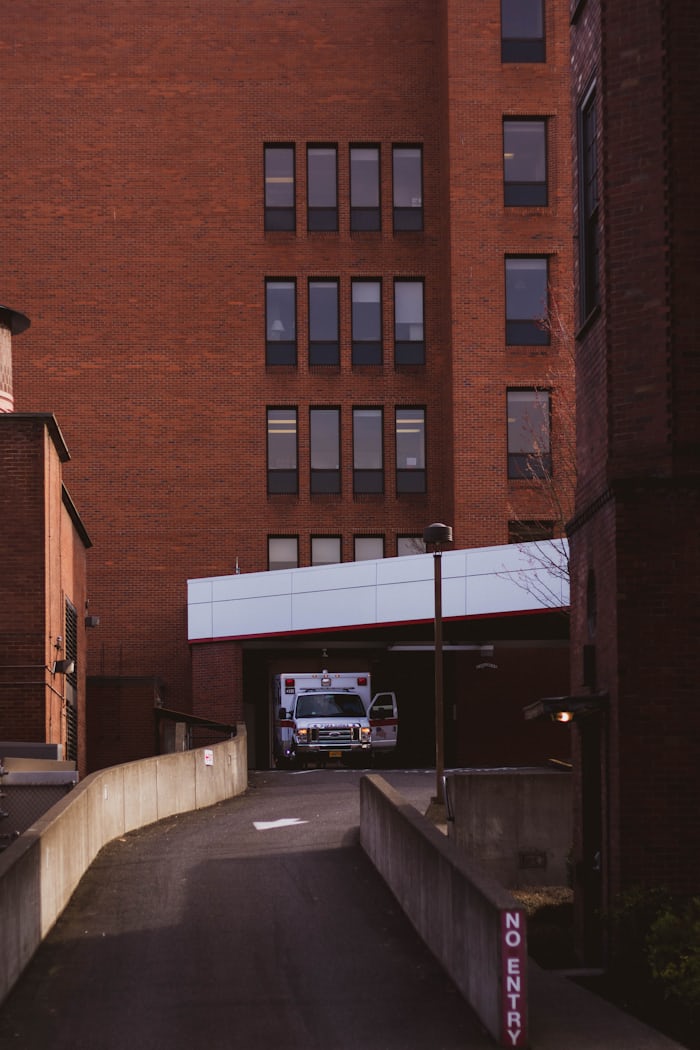
The New Healthcare Math: Why Prevention-Focused AI is a Game-Changer for Payers
Healthcare’s traditional equation has been simple: treat patients when they’re sick. But AI is rewriting this formula by shifting focus to prevention, creating a new economic model that’s catching the attention of insurance companies and healthcare payers worldwide.
Understanding the Cost–Prevention Relationship
Traditional Healthcare
- Reactive treatment costs
- Emergency interventions
- Late-stage disease management
- Higher insurance payouts
AI-Enabled Preventive Care
- Early risk identification
- Proactive interventions
- Lifestyle modification programs
- Reduced serious illness costs
The Insurance Perspective
Insurance companies are particularly interested in AI prevention tools because:
- Early intervention costs less than treating advanced conditions
- Predictive models help in better risk assessment
- Prevention-focused policies can offer lower premiums
- Better data leads to more accurate pricing models
Real Economic Benefits
Several large insurers report:
- 15-20% reduction in hospital admissions through early intervention
- Significant decrease in emergency room visits
- Lower costs for managing chronic conditions
- Better adherence to preventive care schedules
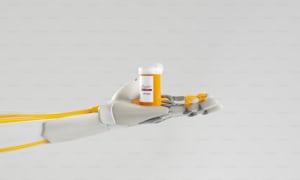
How AI Makes Prevention Possible
1. Risk Prediction
- Analysis of patient data to identify high-risk individuals
- Early warning systems for potential health issues
- Population health trend analysis
2. Personalized Prevention Plans
- Customized health recommendations
- Automated health coaching
- Regular monitoring and adjustments
3. Engagement and Compliance
- Cost Impact Areas
- Reduced emergency care needs
- Lower chronic disease management costs
- Fewer hospital readmissions
- More efficient resource allocation
- Smart reminders for preventive care
- Personalized wellness programs
- Real-time health monitoring
Challenges and Considerations
- Initial investment in AI systems
- Data privacy concerns
- Patient engagement variables
- Integration with existing systems
Future Implications
The shift toward prevention is creating:
- New insurance product models
- Value-based care opportunities
- Different provider compensation structures
- Enhanced focus on patient engagement
This new healthcare math shows that prevention-focused AI isn’t just about better health outcomes – it’s about fundamental economic transformation in healthcare delivery. The key is understanding how to leverage these tools effectively while maintaining patient privacy and engagement.
Beyond the Hype: ROI Metrics That Actually Matter in Healthcare AI
Let’s cut through the buzzwords and focus on the metrics that truly indicate whether AI investments in healthcare are delivering value. While vendors often tout impressive-sounding statistics, experienced healthcare providers know that only certain numbers really matter.
Key Performance Indicators Worth Tracking
1. Time-Based Metrics
- Reduction in patient wait times
- Time saved per clinical procedure
- Staff hours recovered from administrative tasks
- Time to diagnosis. These matter because time directly correlates with costs and patient satisfaction.
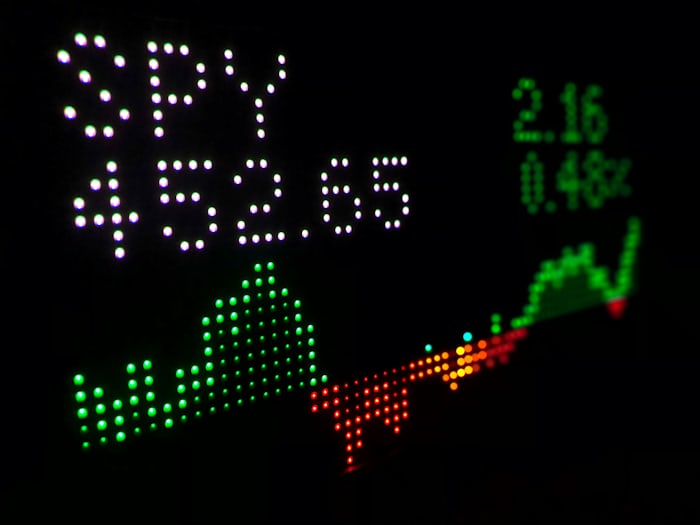
2. Financial Metrics
- Direct cost savings
- Revenue increases from improved efficiency
- Reduction in unnecessary procedures
- Staff overtime costs
- Return on initial AI investment
3. Quality Indicators
- Diagnostic accuracy rates
- False positive/negative rates
- Patient readmission rates
- Clinical outcome improvements
These show whether AI is actually improving care, not just cutting costs.
4. Staff Impact Metrics
- User adoption rates
- Staff satisfaction scores
- Training time required
- Productivity changes
Because AI systems are only valuable if they’re actually being used effectively.
5. Patient-Centered Metrics
- Patient satisfaction scores
- Engagement rates with AI tools
- No-show rates for appointments
- Treatment plan adherence
Real ROI Timeline Expectations
Short-term (6-12 months):
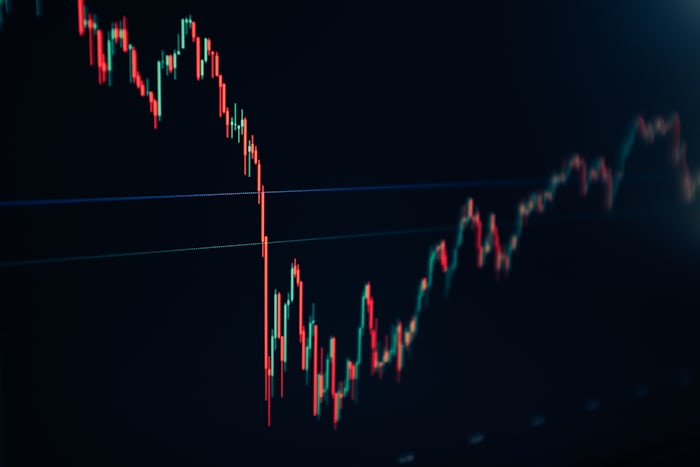
- Reduced administrative workload
- Better scheduling efficiency
- Initial staff time savings
Medium-term (1-2 years):
- Improved diagnostic accuracy
- Reduced operational costs
- Better resource utilization
Long-term (2+ years):
- Significant cost savings
- Better patient outcomes
- Enhanced predictive capabilities
Red Flags to Watch For
- Metrics that can’t be directly tied to business objectives
- Vanity metrics that sound impressive but don’t impact outcomes
- Statistics that ignore implementation and maintenance costs
- Numbers that don’t account for staff training time
Best Practices for Measurement
- Establish clear baselines before implementation
- Use consistent measurement methods
- Track both positive and negative impacts
- Consider indirect effects on workflow
- Measure at regular intervals
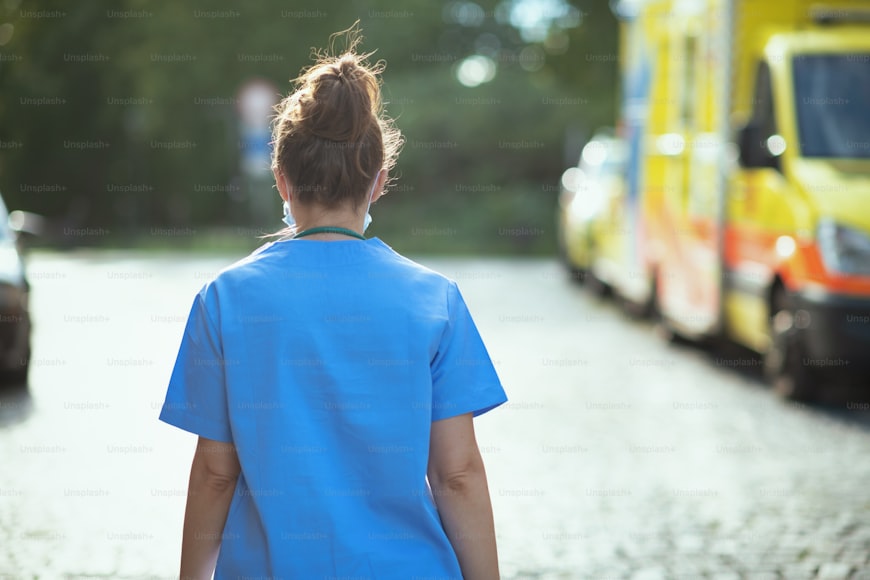
Why Traditional ROI Calculations May Fall Short
Healthcare AI often delivers benefits that don’t fit neatly into traditional ROI calculations:
The most meaningful metrics are those that show:
- Real cost savings
- Genuine improvement in patient care
- Actual efficiency gains
- Sustainable long-term benefits Improved patient experience
- Better work-life balance for staff
- Enhanced reputation
- Future-proofing operations
The Bottom Line
Success in healthcare AI isn’t just about impressive technology – it’s about measurable improvements in care delivery, efficiency, and outcomes.
Remember: The best metrics tell a story about how AI is improving healthcare delivery, not just about the technology itselfself